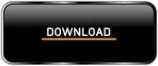
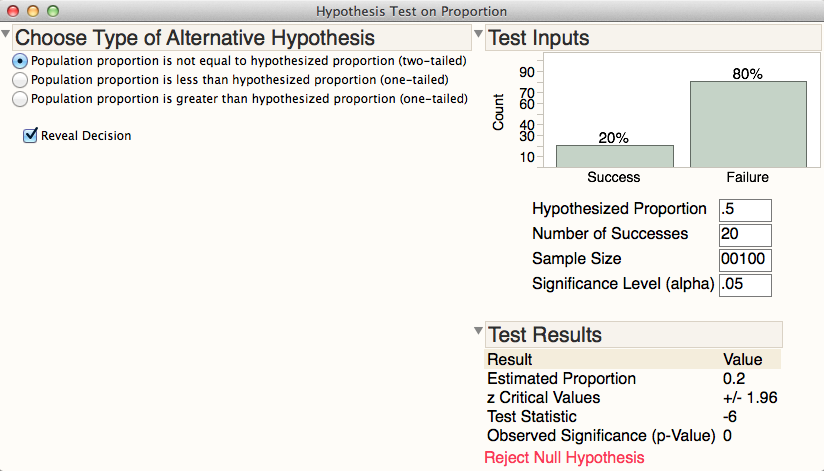
#Paired t test jmp trial#
14.Tanner MA, Galanello R, Dessi C, Smith GC, Westwood MA, Agus A, Roughton M, Assomull R, Nair SV, Walker JM, Pennell DJ (2007) A randomized, placebo-controlled, double-blind trial of the effect of combined therapy with deferoxamine and deferiprone on myocardial iron in thalassemia major using cardiovascular magnetic resonance.14.3 - Measures of Association for Binary Variables.14.2 - Measures of Association for Continuous Variables.13.5 - Obtain the Canonical Coefficients.13.4 - Obtain Estimates of Canonical Correlation.Test for Relationship Between Canonical Variate Pairs 13.1 - Setting the Stage for Canonical Correlation Analysis.Lesson 13: Canonical Correlation Analysis.12.7 - Maximum Likelihood Estimation Method.12.6 - Final Notes about the Principal Component Method.12.4 - Example: Places Rated Data - Principal Component Method.11.7 - Once the Components Are Calculated.11.6 - Example: Places Rated after Standardization.11.5 - Alternative: Standardize the Variables.11.4 - Interpretation of the Principal Components.11.2 - How do we find the coefficients?.11.1 - Principal Component Analysis (PCA) Procedure.Lesson 11: Principal Components Analysis (PCA).10.5 - Estimating Misclassification Probabilities.10.1 - Bayes Rule and Classification Problem.9.6 - Step 3: Test for the main effects of treatments.9.5 - Step 2: Test for treatment by time interactions.9.3 - Some Criticisms about the Split-ANOVA Approach.8.10 - Two-way MANOVA Additive Model and Assumptions.8.9 - Randomized Block Design: Two-way MANOVA.8.7 - Constructing Orthogonal Contrasts.8.4 - Example: Pottery Data - Checking Model Assumptions.8.2 - The Multivariate Approach: One-way Multivariate Analysis of Variance (One-way MANOVA).8.1 - The Univariate Approach: Analysis of Variance (ANOVA).Lesson 8: Multivariate Analysis of Variance (MANOVA).7.2.8 - Simultaneous (1 - α) x 100% Confidence Intervals.7.2.7 - Testing for Equality of Mean Vectors when \(Σ_1 ≠ Σ_2\).7.2.6 - Model Assumptions and Diagnostics Assumptions.7.2.4 - Bonferroni Corrected (1 - α) x 100% Confidence Intervals.7.2.2 - Upon Which Variable do the Swiss Bank Notes Differ? - Two Sample Mean Problem.7.2.1 - Profile Analysis for One Sample Hotelling's T-Square.7.1.15 - The Two-Sample Hotelling's T-Square Test Statistic.7.1.12 - Two-Sample Hotelling's T-Square.7.1.11 - Question 2: Matching Perceptions.7.1.8 - Multivariate Paired Hotelling's T-Square.7.1.7 - Question 1: The Univariate Case.7.1.4 - Example: Women’s Survey Data and Associated Confidence Intervals.7.1.1 - An Application of One-Sample Hotelling’s T-Square.Lesson 7: Inferences Regarding Multivariate Population Mean.6.2 - Example: Wechsler Adult Intelligence Scale.Lesson 6: Multivariate Conditional Distribution and Partial Correlation.5.2 - Interval Estimate of Population Mean.5.1 - Distribution of Sample Mean Vector.Lesson 5: Sample Mean Vector and Sample Correlation and Related Inference Problems.4.7 - Example: Wechsler Adult Intelligence Scale.4.6 - Geometry of the Multivariate Normal Distribution.4.4 - Multivariate Normality and Outliers.4.3 - Exponent of Multivariate Normal Distribution.Lesson 4: Multivariate Normal Distribution.Lesson 3: Graphical Display of Multivariate Data.Lesson 2: Linear Combinations of Random Variables.1.5 - Additional Measures of Dispersion.Lesson 1: Measures of Central Tendency, Dispersion and Association.Next 7.1.16 - Summary of Basic Material ».« Previous 7.1.14 - The Multivariate Case.The counterfeit notes can be distinguished from the genuine notes on at least one of the measurements.Īfter concluding that the counterfeit notes can be distinguished from the genuine notes the next step in our analysis is to determine upon which variables they are different. The sample variance-covariance matrix for the real or genuine notes appears below: The sample mean vectors are copied into the table below:
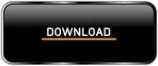